Recent Advances in Microvascular Function Research
Introduction
Microvascular function is essential in maintaining cardiovascular health, adequate blood flow and tissue oxygenation. The diseases that have been linked to dysfunction in microvascular networks include diabetes, hypertension, and heart failure; and it has emerged as an important focus area in current biomedical research. Innovations in both experimental and computational approaches are transforming the way for scientists to understand microvascular dynamics, thereby causing researchers to investigate new diagnostics and therapies for microvascular dysfunction. This article explores the latest research trends in microvascular function, which highlights emerging technologies and interdisciplinary approaches that advance both experimental and computational methodologies.
Key Experimental and computational Approaches in Microvascular Research
-
Imaging Technique
With the development of the latest technique in imaging, it has become easier to visualize microvascular function in real time. Techniques such as confocal, intravital microscopy, and non-invasive modalities such as OCT and MRI, made it possible to view microvascular networks in high resolution [1]. These tools also help in monitoring blood flow, endothelial function, and vessel permeability in live tissues, which is a useful for investigators to understand microvascular pathophysiology.
-
Tissue Engineering and 3D Models
Especially for the purpose of 3D vascular models, tissue engineering is interpreted in the context of its application for mimicking microvascular networks whose intricate structure and function yet support morphology or even functionality [3]. Organ-on-chip technologies and bioengineered scaffolds that mimic the mechanical and biological environment of the microvasculature have been found indispensable for drug testing and personalized medicine [4] [5]. These models help to understand endothelial responses to stress and injury and the effectiveness of therapeutic agents. Moreover, these modeling systems help to reproduce a more complex biological environment precisely compared to traditional systems in vivo animal experiments. However, standardization is one of the biggest challenges in the field of OOCs. Agent-based modeling, is a technique by which the behavior of individual cells can be simulated within the microvascular environment [2]. Various research studies have used agent-based models to address cellular interactions as agents influencing microvascular dynamics, particularly in inflammatory and ischemic conditions.
-
Microfluidics and Lab-on-a-Chip Technologies
Microfluidic devices, often termed “lab-on-a-chip,” serve as controlled models of microvascular environments. These devices are especially useful in studying microvascular networks and the interaction between cells with respect to blood flow and shear stress. This lab-on-a-chip platform enables robust, automated, simplified, and cost-effective point-of-care testing [1]. The key applications include high-throughput drug screening and the vascular response to various stimuli.
-
Computational Fluid Dynamics (CFD)
CFD techniques are used to study blood flow in microcirculation, allowing for detailed examinations of the shear stress and the effects imposed on endothelial function [6]. Modeling blood flow dynamics can explain how dysfunction of the microvasculature contributes to ischemia and other events in the cardiovascular system. These models are particularly useful in predicting the onset and progression of microvascular diseases, thus allowing for targeted interventions.
-
Agent-Based Modeling
This technique emulates the behavior of individual cells in the microvascular environment. Researchers used this agent-based model in research to study the effects of cellular interactions on microvascular dynamics, basically under inflammation and ischemia conditions [2]. ABMs capture heterogeneity and emergent phenomena, leading towards deeper knowledge in a tailored, data-driven fashion of disease processes, treatment responses, and microcirculatory dynamics.
-
Machine Learning and Artificial Intelligence
Machine learning (ML) and artificial intelligence (AI) have dramatically changed the analysis of large amounts of imaging, genetic studies, and patient-monitoring data. AI algorithms can identify patterns and correlations in microvascular data that are simply missed with traditional applications of statistical methods, and these analyses may be helpful in predicting disease progression and tailoring treatment to the individual. A machine learning approach is now helpful in predicting hemodynamic variables in 3D with high accuracy, using artificial neural networks and convolution-based U-net models. The models are helpful in predicting flow, RBC distribution, blood velocity, concentration profiles, and time-dependent variations in blood flow [7]. Further, recent machine learning models are showing promising results in predicting sub-cellular resolved capillary hemodynamics in organ-scale networks through image-based predictions.
-
Integration of Omics Data
Computational models are integrated with data on the genomic, proteomic, and metabolic levels. These approaches will lead to the identification of new biomarkers and therapeutic targets for CMD. They give a very complete view of the molecular landscape associated with microvascular dysfunction [9]. Recently, omics data and machine learning have been used in the clinic setting to identify biomarkers for mental illness, predict who will fail treatment, classify subgroups of disorder, and make data-driven distinctions between patients.
Conclusion
Experimental and computational advances are changing the landscape of microvascular research fast. These technologies offer a new understanding of microvascular function, thus potentially personalizing therapies for microvascular diseases. Further, integration of these approaches, together with personalized medicine and emerging technologies, holds promise for improving the diagnosis and treatment of microvascular disorders. Continued collaboration and innovation are in demand to overcome these challenges in this critical area of cardiovascular health.
References
- Raut, S.S., Acharya, S., Deolikar, V. and Mahajan, S., 2024. Navigating the frontier: emerging techniques for detecting microvascular complications in type 2 diabetes mellitus: a comprehensive review. Cureus, 16(1).
- Coccarelli, A. and Nelson, M.D. (2023) Modeling reactive hyperemia to Better Understand and assess microvascular function: a review of techniques. Annals of Biomedical Engineering, 51(3), pp.479-492.
- Kim, J.J. and Cho, D.W. (2024) Advanced strategies in 3D bioprinting for vascular tissue engineering and disease modelling using smart bioinks. Virtual and Physical Prototyping, 19(1), p.e2395470.
- Tolabi, H., Davari, N., Khajehmohammadi, M., Malektaj, H., Nazemi, K., Vahedi, S., Ghalandari, B., Reis, R.L., Ghorbani, F. and Oliveira, J.M. (2023) Progress of microfluidic hydrogel‐based scaffolds and organ‐on‐chips for the cartilage tissue engineering. Advanced Materials, 35(26), p.2208852.
- Lee, J., Park, J., Kim, S., Han, E., Maeng, S. and Han, J. (2024) Current and Future Perspectives of Lung Organoid and Lung-on-chip in Biomedical and Pharmaceutical Applications. Journal of Life Science, 34(5), pp.339-355.
- Rehan, R., Yong, A., Ng, M., Weaver, J. and Puranik, R. (2023) Coronary microvascular dysfunction: a review of recent progress and clinical implications. Frontiers in Cardiovascular Medicine, 10, p.1111721.
- Ebrahimi, S. and Bagchi, P. (2024) Machine learning techniques for predicting microvascular network hemodynamics. Physiology, 39(S1), p.791.
- Haase, M., Comlekoglu, T., Petrucciani, A., Peirce, S.M. and Blemker, S.S. (2024) Agent-based model demonstrates the impact of nonlinear, complex interactions between cytokinces on muscle regeneration. Elife, 13, p.RP91924.
- Sathyanarayanan, A., Mueller, T.T., Moni, M.A., Schueler, K., Baune, B.T., Lio, P., Mehta, D., Baune, B.T., Dierssen, M., Ebert, B. and Fabbri, C. (2023) Multi-omics data integration methods and their applications in psychiatric disorders. European Neuropsychopharmacology, 69, pp.26-46.
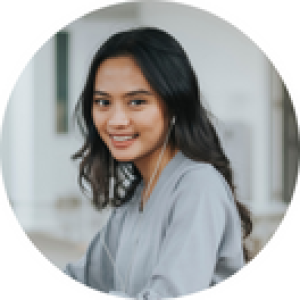
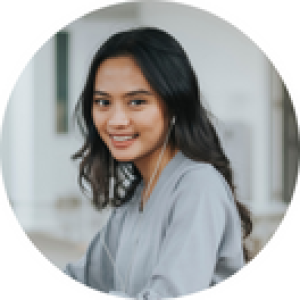