
A Predictive and Qualitative AI Medical Data Collection in Pregnancy Health
October 6, 2023
How Randomized Controlled Trials are Used in Meta-Analysis
October 26, 2023A Systematic Review of Machine Learning Methods for Predicting Cardiovascular Disease
A Systematic Review of Machine Learning Methods for Predicting Cardiovascular Disease
Introduction
Heart diseases are a major global health problem, accounting for 17.7 million deaths per year and 31% of all fatalities worldwide. They have become the top cause of death in India, accounting for 1.7 million fatalities in 2016. These diseases increase healthcare spending and reduce productivity, with estimates suggesting India lost up to $237 billion due to heart-related diseases. Artificial Intelligence and Machine Learning techniques can help predict the presence or absence of heart-related diseases accurately. The increasing use of information technology in healthcare aids doctors in decision-making, disease management, and discovery of patterns among diagnosis data. Current literature review and systematic review approaches to predicting cardiovascular risk often fail to identify those who would benefit from preventive treatment or unnecessary intervention.
Literature Survey
The writers found that the support vector machine (SVM) achieved maximum accuracy of 79.12% with 37 number splits and 6 leaf nodes, while the decision tree achieved 79.54% with a 5-fold. Random forest algorithm, which uses decision trees for voting and classification, achieved an accuracy of 85.81% with 20 splits, 75 trees, and 10 folds.
Dataset
The Heart dataset, available in a Machine Learning Repository, contains 303 samples and 14 input features describing loan applicants’ financial, personal, and social aspects. It consists of 700 instances of good credit and 300 instances of bad credit.
Random Forest
Random Forest is a supervised machine learning method that excels at classification tasks by taking into account several decision trees before producing an output. In classification, it employs a voting method, and in regression, it employs the mean of all decision tree outputs.
Support vector machines
Support vector machines (SVM) are linear and non-classifier classifiers used in training and testing datasets. They are popular due to their good empirical performance and ability to make errors. SVM classifiers are used in many applications and are preferred over naive Bayes in recent research.
Artificial Neural Network
Neural networks (ANN) are used to simulate neural systems, syncing modules in layers. They are built by stacking multiple neurons in layers, with input and output layers. Each neuron has an activation function, and the network’s parameters are weights and biases. The goal is to learn network parameters so the predicted outcome matches the ground truth, using backpropagation along the loss function.
Comparison with Traditional Risk Assessment Models
Evaluate the performance of machine learning models with those of classic risk assessment techniques like the Framingham Risk Score or SCORE. Emphasize the potential benefits of machine learning, such as increased accuracy and personalized risk prediction.
To know more about research proposal services, check our study guide on a Step-by-Step Guide to develop an effective research proposal.
Discussion
Support vector machine, artificial neural network, and random forest data categorization techniques were used. The project entailed analyzing the heart disease patient dataset and properly processing the data. The following models were then trained and tested with the highest possible scores:
- Support Vector Classifier: 84.0 %
- Neural Network: 83.5 %
- Random Forest Classifier: 80.0 %
Conclusion
In conclusion, this systematic review underscores the growing role of machine learning in cardiovascular disease risk assessment. Machine learning algorithms exhibit promise in enhancing risk prediction accuracy, offering a dynamic and personalized approach to cardiovascular health. While various algorithms and datasets have been explored, there is a need for standardized data sources and evaluation metrics to facilitate direct comparisons. Moreover, the integration of these advanced models into clinical practice holds tremendous potential for early intervention and improved patient outcomes. As we move forward, addressing data limitations and ensuring model interpretability are crucial challenges. Nonetheless, the evolving landscape of machine learning in cardiovascular care opens new avenues for preventing and managing heart diseases.
- Check our Examples to get an understanding of our adaptability across topics and subject domains.
About Pubrica
Pubrica’s team of researchers and authors develop Scientific and medical research services that can act as an indispensable tool to the practitioner/authors. Our systematic review at Pubrica is more structured at every stage of writing, and we ensure to critically check the rigour using standard methodological checklists such as PRISMA, CASP, AMSTAR, and ARIF, etc., based on the checklist provided. Our experts are aware of the structure that follows the broad topic, the problem, and the background and advance to a narrow topic to state the hypothesis.
References
- Azmi, Javed, et al. “A systematic review on machine learning approaches for cardiovascular disease prediction using medical big data.” Medical Engineering & Physics 105 (2022): 103825.
- Rindhe, Baban U., et al. “Heart disease prediction using machine learning.” Heart Disease 5.1 (2021).
- Islam, Saiful, Nusrat Jahan, and Mst Eshita Khatun. “Cardiovascular disease forecast using machine learning paradigms.” 2020 Fourth International Conference on Computing Methodologies and Communication (ICCMC). IEEE, 2020.
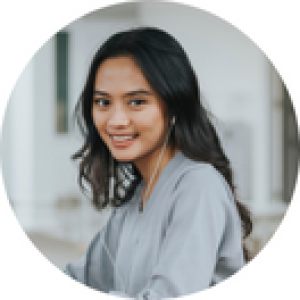
14 years of expertise in clinical research with a doctoral distinction in life science.
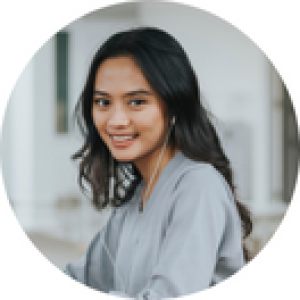