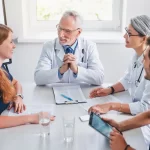
How healthcare professionals can use EBP in assessment & diagnosis
June 11, 2021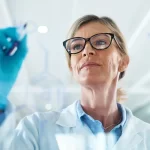
How Evidence-based practice (EBP) can be translated as health communication or patient education materials
June 15, 2021Introduction
Effect size is a statistical idea that helps measure the strength and connection between two variables on a numeric scale.
It simply refers to the size and the difference found between the two groups. It’s simple to compute, understand, and apply to any educational or social science outcome that can be quantified. It’s especially useful for calculating the efficiency of a certain intervention concerning other interventions.

It is useful for calculating the efficiency of a certain intervention in relation to other interventions. It enables us to look further from the simple ‘Does it function or not?’ question to “How well does it work in a variety of contexts?” and significantly more complex, by focusing on the most crucial feature of an intervention. Rather than its statistical significance, it promotes a different scientific approach to the accumulation of knowledge. For these reasons, the effect size is considered an effective tool in reporting and interpreting effectiveness.
For example, if we have data on the weight of men and women and notice that, on average, men have more weight than women, women’s weight is known as the effect size.
Statistical effect size helps us decide whether the difference is genuine or a difference in factors. [1]
SIGNIFICANCE OF EFFECT SIZE:
Formulae for evaluating the effect sizes do not often found in many statistics textbooks (other than those devoted to meta-analysis), are not included in various statistics computer packages and are occasionally taught in standard research approaches courses. For these above-stated reasons, even the researcher who found interest in using measures of effect size is afraid to use them in conventional practice and find it quite hard to know exactly how to do it.
EFFECT SIZE IN META-ANALYSIS
In Meta-analysis, the effect size is concerned about various studies and afterwards joins all the studies into a single analysis.[2]
In statistical analysis, the effect size is typically estimated in three ways:
(1) The standardized mean difference,
(2) Odd ratio,
(3) Correlation coefficient.
FORMULATION FOR EFFECT SIZE:
Karl Pearson created Pearson r correlation, and it is broadly utilized in statistics.[3] This parameter of effect size is signified by r—the estimation of the effect size of the Pearson r connection shifts is found in-between -1 to +1.

Where
r = correlation coefficient
N = number of pairs of scores
∑XY = sum of the products of paired scores
∑x = sum of x scores
∑y = sum of y scores
∑x2= sum of squared x scores
∑y2= sum of squared y scores
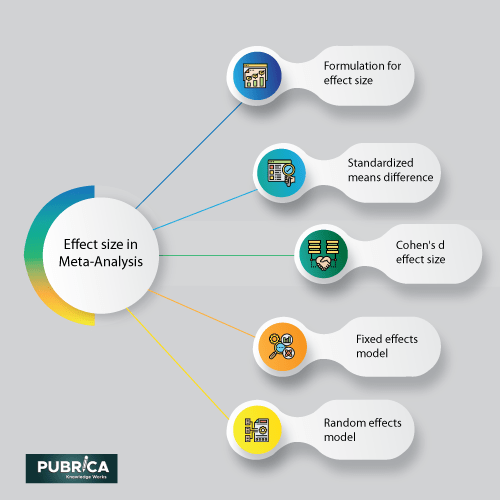
Standardized means difference:
When a research study depends on the population mean and standard deviation, at that point, the accompanying technique is utilized to know the effect size:
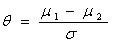
Cohen’s d effect size:
Cohen’s d is known as the distinction of two population means, and the standard deviation separates it from the data. [4]
Mathematically Cohen’s effect size is signified by:
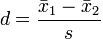
Where s can be calculated by using the following formula:
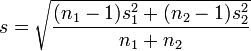
Hedges’ g method of effect size: This is the modified form of Cohen’s d method. We can write Hedges’ g method of effect size as follows:
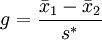
FIXED EFFECTS MODEL:
The fixed-effect model gives a weighted average of a progression of study estimates. The opposite of the appraisals’ difference is usually utilized as study weight. More extensive studies will offer more than smaller studies to the weighted average. Thus, when concentrates inside a meta-analysis are overwhelmed by an extensive study, the discoveries from smaller studies are practically ignored. [7]
This assumption is ordinarily unrealistic as an examination is frequently inclined to several heterogeneity sources; for example, treatment impacts may contrast as indicated by region, measurements levels, and study conditions.
RANDOM EFFECTS MODEL:
A typical model used to synthesize heterogeneous study is the irregular impacts model of meta-analysis. This is the weighted average of the effect sizes of a gathering of studies. The weight that is applied in this interaction of weighted averaging with an arbitrary impacts meta-investigation is accomplished in two stages:
Step 1: Inverse variance weighting.
Step 2: Un-weighting of inverse variance weighting by REVC (Random Effects Variance Component).
CONCLUSION AND FUTURE ENHANCEMENTS:
The more significant variability in effect size e (also called heterogeneity) is the more prominent in un-weighting.
This can conclude that the arbitrary impacts meta-analysis result turns out to be just the un-weighted average effect size across the studies. At the other limit, when all effect sizes are comparable (or inconstancy doesn’t surpass testing error), no REVC is applied, and the irregular impacts meta-examination defaults to just a fixed impact meta-investigation (just opposite variance weighting).
References:
[1] Ens, D. (2013). Calculating and reporting effect sizes to facilitate cumulative science: a practical primer for t-tests and ANOVAs. Front. Psychol. 4:863. doi: 10.3389/fpsyg.2013.00863
[2] Assen, M. A. L. M., van Aert, R. C. M., and Wicherts, J. M. (2015). Meta-analysis using effect size distributions of only statistically significant studies. Psychol. Methods 20, 293–309. doi: 10.1037/met0000025
[3] Gavin Brupbacher, Heike Gerger, Thea Zander-Schellenberg, Doris Straus, HildburgPorschke, Markus Gerber, Roland vonKänel, Arno Schmidt-Trucksäss, The effects of exercise on sleep in unipolar depression: A systematic review and network meta-analysis,Sleep Medicine Reviews, (2021).
[4] Behm, D.G., Alizadeh, S., Anvar, S.H. et al. Non-local Acute Passive Stretching Effects on Range of Motion in Healthy Adults: A Systematic Review with Meta-analysis. Sports Med (2021).
[5] Nicholas Clarke, Lars PødenphantKiær, O. JanneKjønaas, Teresa G. Bárcena, Lars Vesterdal, Inge Stupak, LeenaFinér, Staffan Jacobson, KęstutisArmolaitis, DagnijaLazdina, Helena Marta Stefánsdóttir, Bjarni D. Sigurdsson,
[6] Effects of intensive biomass harvesting on forest soils in the Nordic countries and the UK: A meta-analysis,
[7] Forest Ecology and Management, (2021).