
Application Of Evidence-Based Medicine in Individualized Immune-Oncology
September 23, 2023
A Systematic Review of Machine Learning Methods for Predicting Cardiovascular Disease
October 12, 2023A Predictive and Qualitative AI Medical Data Collection in Pregnancy Health
A Predictive and Qualitative AI Medical Data Collection in Pregnancy Health
Table of Content
Introduction
Artificial Intelligence (AI) has gained significant interest in healthcare and medicine, particularly in predicting health-related problems like diabetes, cardiovascular diseases, obesity, cancer, and pregnancy disorders. Prediction studies can be categorized into risk factor studies, prediction models studies, and prediction models impact studies. These studies aim to identify predictors, develop, Medical Data collection, validate, or improve prediction models for personalized patient care, and evaluate the impact of using these models.
- Check our Examples to get an understanding of our versatility across topics and subject domains.
Medical Data Collection
- Medical Records: Gather historical medical records and data related to the expectant mother’s health, including past pregnancies, medical conditions, and genetic history.
- Biometric Data: Collect real-time biometric data such as heart rate, blood pressure, and weight through wearable medical devices or home monitoring equipment.
- Lifestyle Data: Gather qualitative data on lifestyle factors, including diet, exercise, stress levels, and sleep patterns through regular surveys or connected apps.
Data Integration
- Integrate all collected data into a centralized database or cloud platform, ensuring data security and compliance with privacy regulations like HIPAA (in the United States) or GDPR (in Europe).
Predictive Analytics
- Utilize machine learning algorithms to analyze historical and real-time data to predict potential health risks or complications during pregnancy.
- Predictive models can provide early warnings for conditions like gestational diabetes, pre-eclampsia, or preterm labor, allowing for timely intervention.
Personalized Guidance
- Develop AI-driven systems that provide personalized guidance to expectant mothers based on their unique health profile.
- These recommendations may include dietary advice, exercise routines, stress management techniques, and reminders for prenatal appointments.
Communication and Support
- Implement chatbots or virtual assistants to offer immediate responses to common pregnancy-related questions.
- Enable secure communication channels for expectant mothers to connect with healthcare professionals, ask questions, and share concerns.
Qualitative Data Collection
- Regularly collect qualitative data through surveys, interviews, or chatbot interactions to understand the emotional and psychological well-being of expectant mothers.
- Analyze this qualitative data to identify stressors, anxiety triggers, and support needs.
Continuous Monitoring
- Maintain continuous monitoring throughout the pregnancy, adapting predictions and recommendations as the mother’s health and circumstances evolve.
Research and Improvement
- Continuously update and improve the Artificial Intelligence system based on new research findings and user feedback to enhance its predictive accuracy and support capabilities.
AI data collection systems in pregnancy health can enhance maternal care by providing personalized guidance, early warning of complications, and emotional support. However, privacy concerns and maintaining a strong feedback loop with medical professionals are crucial for data security and quality care.
- To know more about medical data collection services, check our study guide on What are the methods and techniques of data collection in health care research
AI Applications in Health and Data Management Technique in a Diverse Environment
- AI has several benefits to healthcare services and can considerably improve patients’ health and well-being. Jiang’s analysis of AI in healthcare’s past, present, and future emphasizes the relevance of clinical data acquired from many sources (medical records in various databases and wearable health monitors). The availability of this data, together with advancements in processing power, simplifies and improves the application of AI in the medical area.
- Its applications include decreasing diagnostic and therapeutic mistakes in clinical practice, as well as gathering relevant information from huge patient populations to aid in drawing real-time inferences for health risk warning and health outcome prediction. AI’s influence on health care has been classified into three categories: it may aid physicians in medical diagnosis through speedy, accurate picture interpretation; it can enhance health system processes and perhaps minimize medical mistakes; and it can empower people to promote their own health.
- AI, combined with advances in information and communications technology, is dramatically altering the experience of consumers for whom technical solutions are developed. The employment of AI in its numerous versions allows for the personalization of technical solutions, enabling major advancements in enhancing the quality of life of patients of various types.
Conclusion
The qualitative study confirms the hypothesis that a pregnant-centered intelligent solution should provide emotional support to prevent pregnancy risks. Women with high-risk pregnancies are more interested in such solutions. Public healthcare systems are considered more trustworthy for pregnancy health and well-being. The study’s findings will guide the next phase of the project, which will use affective computing and wearable IoT to validate the methodology in a pregnancy case study.About Pubrica
At Pubrica, we collect data from a wide range of sources and perform semantic annotation based on the research questions that you wanted to solve. Pubrica has the vast majority of the data in doctor’s notes; electronic medical records, prescriptions, and similar information are available. Although therein lies the golden possibility of big data in medical care, it’s challenging to yield valuable insights due to complex, unstructured, longitudinal, and voluminous data.References
- Oprescu, Andreea M., et al. “Towards a data collection methodology for Responsible Artificial Intelligence in health: A prospective and qualitative study in pregnancy.” Information Fusion 83 (2022): 53-78.
- Delanerolle, Gayathri, et al. “Artificial intelligence: a rapid case for advancement in the personalization of gynaecology/obstetric and mental health care.” Women’s Health 17 (2021): 17455065211018111.
- Lau, Annie YS, and Pascal Staccini. “Artificial intelligence in health: new opportunities, challenges, and practical implications.” Yearbook of medical informatics 28.01 (2019): 174-178.
Table of Content
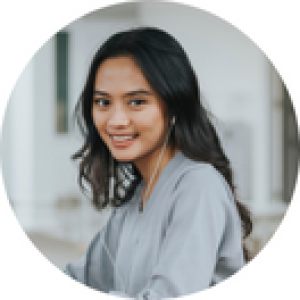
14 years of expertise in clinical research with a doctoral distinction in life science.
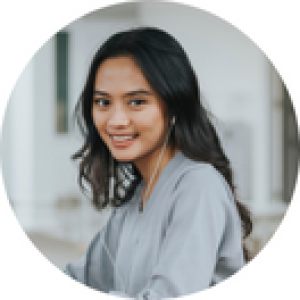