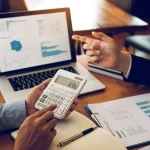
Evidence-based analyses to look at cost-effectiveness, cost-benefit information & clinical data from RT – Device manufacturers
May 18, 2021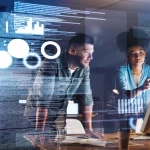
What are the different sources of data used to extract for writing a systematic review
May 26, 2021Introduction
In healthcare studies, systematic reviews are valuable sources of evidence. These are regarded as having a high degree of evidence because they reduce bias during the evaluation process, offer detailed evidence regarding the efficacy of an experiment, and often resolve uncertainty caused by contradictory findings from various researches asking the same issue. Meta-analysis is an effective computational method for obtaining a single effect size by combining the outcomes of multiple individual experiments. As a result, the best level of proof is known to be a systematic study with meta-analysis.
The conventional meta-analysis approach does not take into account previous knowledge from outside sources. As a result, a new approach to meta-analysis is established, in which historical data is combined using Bayesian principles. “The clear comparative use of external data in the design, control, study, and understanding of health care evaluation,” according to the Bayesian approach. The prior belief about the parameter, which should be external to data, is one of the criteria of Bayesian meta-analysis. The observed data were paired with prior experience to provide new information about the parameter of interest [1].
The focus of this article is to define how extensively Bayesian methods have been used in meta-analysis, benefits, and implementations.
BAYESIAN METHODS: THE PRINCIPLES
Standard statistical inference means that the sample comes from a population with a fixed and undefined parameter. The sample information is used to make the whole parameter inference. On the other hand, the Bayesian method treats parameters as random variables with a probability distribution that reflects our prior knowledge. The likelihood function is summarised in the current data. The prior distribution and probability function was merged using Bayesian rules to produce the posterior distribution function [2].
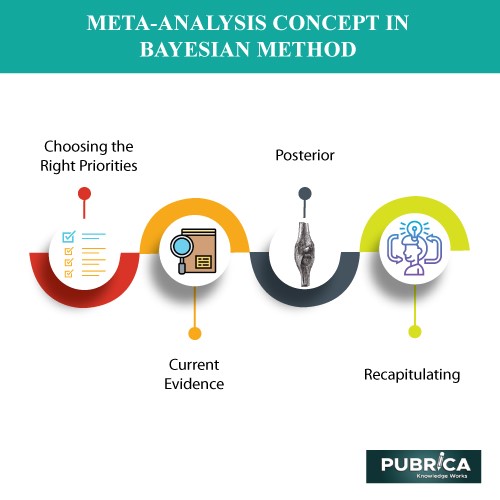
META-ANALYSIS CONCEPT IN BAYESIAN METHOD
There are four basic stages in a Bayesian meta-analysis [2]:
(1) Choosing the Right Priorities
The first step in Bayesian meta-analysis is to summarise the proof that isn’t based on observed facts. This document reviews previous evidence and assumptions about intervention’s relative benefits. Non-randomized experiments, invitro or invivo trials, experimental studies, or personal views may be used as verification. Since the parameters are called unpredictable random variables, prior distributions are applied to them.
(2) Current Evidence
The probability function of the parameters would be composed of observable data or impact predictions gathered from various studies asking the same query. For both measurable and unobservable quantities, a complete probability model is constructed.
(3) Posterior
The external information is then combined with the current data to arrive at a current understanding of the intervention’s impact. As a result, the posterior distribution is derived by combining the prior distribution and the probability function. The revised proof is another name for the posterior. In addition, unlike conventional Meta-analysis, all inferences should be based on the posterior distribution.
(4) Recapitulating
In Bayesian Meta-analysis, the final step is to summarise the posterior distribution. The posterior distribution obtained is often of high dimension and complexity, necessitating computer-based packages (BUGS and WINBUGS) to execute the integrations. Simulation techniques like Markov Chain Monte Carlo are used to sample directly from the posterior distribution. As a result, all summary figures, such as mean, standard deviation, odds ratio, risk ratio, and so on, are calculated using those samples. Instead of 95 percent confidence intervals, 95 percent accurate intervals (2.5 percentile and 97.5 percentile of posterior distribution) were measured. In Bayesian meta-analysis, two methods are widely used, similar to conventional meta-analysis: fixed-effect and random-effects models. The only difference between Bayesian Meta-analysis and conventional meta-analysis is that prior distributions for uncertain parameters are defined.
BAYESIAN META-ANALYSIS PROFITS AND CONTRAINDICATIONS
In prior distribution, Bayesian meta-analysis integrates all applicable historical data outside of the litigation. They account for all uncertainties, especially when determining a predictive distribution for the true effect in a new sample. When there are a limited number of studies involved, or when studies have fewer case results, or when studies report only the summary estimation rather than its variance, Bayesian meta-analysis is sufficient. The posterior distribution is optimal for any decision-making situation [3], and the odds are more understandable than p values. They also provide for the interpretation of the likelihood or consequence of action. Prior probabilities can be used as a sensitivity analysis [4] instrument to search for robustness andanalyses and calculate various theories [5]. The main drawback is that as the number of parameters increases with the number of experiments, imposing vague priors on all parameters will lead to contradictory outcomes. Different prior distributions provide different outcomes. Researchers must exercise caution when using informative priors since they may significantly affect the posterior. The software’s implementation necessitates excellence.
FUTURE SCOPE
Due to Bayesian’s clear methodology for integrating external data, these approaches are commonly used in network meta-analysis. One renders both direct and indirect observations dependent on a generic comparator and ranks interventions. However, although software makes much of the work simpler, it still necessitates many computational assistance and skills. In the field of clinical trial proof synthesis, Bayesian meta-analysis has gained attention. Because public health interventions are geared to geographically heterogeneous demographic, multi-component interventions, context-specific, and various effects, it did not gain traction in summarising them. The use of conventional meta-analysis to combine the findings of such analyses has not been thoroughly studied. A recent effort was made to investigate the complexities of public health approaches and create a meta-analysis for public health interventions that took complexity into account. Any public health intervention’s data is typically obtained from a mixture of retrospective and interventional trials. Since there is no common mechanism for combining the findings of retrospective and intervention trials, most systematic analyses are presented narratively. As a result, in complicated public health research, a reliable method of evidence synthesis is needed. Finally, Bayesian meta-analysis-specific reporting criteria must be established.
Reference:
[1] Lewis, Melissa Glenda and Nair, Sreekumaran N (2015) Review of applications of Bayesian meta-analysis in systematic reviews. Global Journal of Medicine and Public Health, 4 (1). pp. 1-9. ISSN 2277-9604.
[2] MatthaisE,GeorgeDS,JonathanAC(2002).Systematicreviews and Meta-‐analysis. In: Oxford textbook of Public Health.4thed. New York: Oxford University press.
[3]Młynarczyk, Dorota; Armero, Carmen; Gómez-Rubio, Virgilio; Puig, Pedro. 2021. “Bayesian Analysis of Population Health Data” Mathematics 9, no. 5: 577..
[4]Mojtaba GANJALI, Taban BAGHFALAKI, Adeniyi Francis FAGBAMIGBE “A Bayesian sensitivity analysis of the effect of different random effects distributions on growth curve models,” AfrikaStatistika, Afr. Stat. 15(3), 2387-2393, (July 2020)
[5]Paulewicz, B., Blaut, A. The bhsdtr package: a general-purpose method of Bayesian inference for signal detection theory models. Behav Res 52, 2122–2141 (2020)..