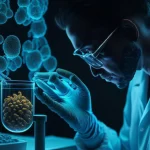
Current Developments in Bioinformatics and Computational Biology
March 5, 2021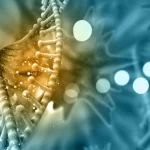
Gene Therapy in Clinical Research Organization
March 9, 2021Effective Strategies to Monitor Clinical Risks using Biostatistics
Effective Strategies to Monitor Clinical Risks Using Biostatistics
In Brief
- In clinical science, biostatistics services are essential for data collection, analysis, presentation, and interpretation. Epidemiology, clinical trials, population genetics, systems biology, and other disciplines all benefit from it. It aids in the evaluation of a drug’s effectiveness and safety in clinical trials.
Introduction
Through quantitative analysis, biostatisticians play a unique role in protecting public health and enhancing people’s lives. Biostatisticians may work with other biomedical experts to find and address issues that threaten health and quality of life by integrating quantitative disciplines. Biostatistician and Statistical Programming devise innovative approaches to ensure that interventions are focused on proof of benefit—whether tailored to communities or people in need of care—from determining the health effects of air pollution to planning and testing new cancer research. Specific patients are examined and treated by clinicians. Understanding the health problems they’ll face, the possible history and potential courses of the clinical issues they’re seeing, and assessing the efficacy and risks of their clinical decisions and interventions are also dependent on client characteristics and histories. Similarly, the person they see right now and with whom they may be about to interfere. Biostatistics in clinical trials is a vital instrument for connecting the various potentials.
Strategies to monitor clinical risks using biostatistics
Biological and clinical entities are multi-dimensional, dynamic, and evolving mechanisms and processes that change over time. Both research projects begin with selecting specific physical objects and process segments that could reflect specific structures and processes in the research.
1) Measurement scaling
Specific dimensions of measuring and sampling are crucial in determining which methodological methods to use. The scaling of the measurements was treated as variables in the study is the first feature that indicates the appropriateness of and thus guides the choice among statistical procedures. Scales are used in statistics to describe measurements. Nominal, ordinal, and interval scalings are used to classify measurements. For each type of observation, nominal scalings use distinct and mutually exclusive numbers. Nominal scalings are only used to categorise observations. No additional knowledge about magnitude is conveyed by the numbers allocated on a nominal scale.
2) Descriptive statistics and measurement scaling: Single variables
Descriptive biostatistics in clinical research describes the fundamental trend, the single best explanation of the sample of observations, and uncertainty in single variable studies. In the analysis, descriptive statistics for single variables play an essential role. In randomised experiments, descriptive statistics outline the traits of the sample and control groups. When comparing nominally sized variables like gender, the proportions are analysed to determine the baseline comparability between an investigation’s sample and control categories. When comparing the ordinally scaled urgency, the median may be used. Averages may be studied when comparing intervally scaled traits, such as group members’ age, serum albumin, and platelet count. And other critical hematologic indices.
3) Descriptive statistics and measurement scaling: Multiple variables
Correlation coefficients typically range from “0”, indicating no association to “−1” and “1”, indicating perfect association. The correlation coefficient’s square can be thought of as the proportion of one variable’s variance estimated by the other. The square of “1” equals the square of “−1” equals “1,” indicating perfect association. For nominal variables, phi and Cramer’s V, Spearman’s rho (or rank-order) correlation for ordinal variables, and Pearson’s r (or product-moment) correlation for interval variables are the most commonly used correlation coefficients. For binomial nominal variables, Kappa is often used. Gender and the occurrence versus absence of a trait or condition are examples of binomial variables with only two values.
4) Measurement timing
Clinical biostatics services and research data and testing results are often collected over a short period as the systems receiving clinical scrutiny and those that are being analysed persist beyond that time frame’s borders. To overcome the challenges posed by what is known as “right censoring,” survival analysis and life-table statistics strategies have been developed. When a study investigates a procedure that has concluded some, but not all, of the topics when the study concludes, right censoring occurs, resulting in censoring facts about the outcome.
5) Modelling associations and prediction
The type of regression modelling that is suitable is determined by the dependent variable’s estimation and completeness. If the dependent variable is a binomial, that is, a minimal variable with just two values, and the result was determined for each member of the sample. Multiple logistic regression was used to predict the independent variables’ influence on the probability ratio of achieving the result. These probability ratios can be treated as measures of each independent variable’s relative likelihood when the outcome scenario is relatively typical and other restrictions encountered.
Conclusion:
Clinicians work with particular patients, but decisions on treatment procedures nearly often consider facets of health courses that certain people have taken. One of the most suitable methods for bridging this distance is statistics. The statistical approach to health incidents and treatment has analysed in this article regarding a few main aspects. The experiments used as models are both scientifically and methodologically sound. However, there are some aspects of the architecture and implementation that methodological flaws have plagued. In light of the sampling and calculations, these include statistical power analysis and sample size preparation and the collection and execution of relevant studies.
References:
- Wulff HR, Andersen B, Brandenhoff P, Guttler F. What do doctors know about statistics? Stat Med. 1987;6:3–10
- Berwick DM, Fineberg HV, Weinstein MC. When doctors meet numbers. Am J Med. 1981;71:991–8.
- Weiss ST, Samet JM. An assessment of physician knowledge of epidemiology and biostatistics. J Med Educ. 1980;55:692–7
- Best AM, Laskin DM. Oral and maxillofacial surgery residents have poor understanding of biostatistics. J Oral Maxillofac Surg. 2013;71:227–34.
- Bookstaver PB, Miller AD, Felder TM, Tice DL, Norris LB, Sutton SS. Assessing pharmacy residents’ knowledge of biostatistics and research study design. Ann Pharmacother. 2012;46:991–9